It’s too soon to trust AI with healthcare

• AI in medicine is highly contentious, despite some impressive results.
• In a for-profit healthcare system, speed to market will always beat extensive testing.
• The FDA has faced criticism for its approval procedures.
READ NEXT
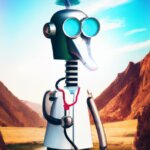
The future of healthcare technology
AI in medicine has been kept, pretty much, on the sidelines: as a scribe, a second opinion and back-office organizer. That’s partly due to the cautionary tales borne of attempts to mix medicine and AI.
One program intended to improve follow-up care for patients deepened troubling health disparities, and another triggered a litany of false alarms when used to detect sepsis.
But of course, no industry wants to miss the AI hype train, and healthcare is no exception. As the technology has improved, gaining investment and momentum for uses in medicine, it’s become a hot topic for the Food and Drug Administration (FDA) in America. Further, the link between medicine and technology is huge and net positive, so it makes sense that many are keen to welcome the tech du jour into the sector.
The up and downsides of AI in medicine
AI is helping discover new drugs, pinpointing unexpected side effects, and being discussed as an aid for staff who are overwhelmed with repetitive, rote tasks. One of these gives many observers significant pause, while the other makes some sense – you can probably guess which is which. The degree to which AI is allowed to deliver “hardcore” medical services is akin to the degree of discomfort it generates in many who, just a year ago, lived in a world without generative AI being added to every industry.
The FDA too has received criticism over how carefully it vets and describes the programs it approves.
“We’re going to have a lot of choices. It’s exciting,” Dr. Jesse Ehrenfeld, president of the American Medical Association said in an interview. “But if physicians are going to incorporate these things into their workflow, if they’re going to pay for them and if they’re going to use them — we’re going to have to have some confidence that these tools work.”
US President Joe Biden issued an executive order on Monday, October 31st, calling for regulations across a broad spectrum of agencies to manage the security and privacy risks of AI, including in healthcare.
The order calls for more funding for AI research in medicine and also for a safety program to gather reports on harmful or unsafe practices.
Google’s pilot of a chatbot for healthcare workers, Med-PaLM 2, drew Congress’ attention recently, raising concerns about patient privacy and informed consent. This follows in the wake of several cases of patient data being compromised not by generative AI, but by something as simple as the Meta Pixel.
How the FDA will oversee such “large language models” — programs that mimic expert advisers — is just one area where the agency lags behind rapidly evolving advances in the AI field.
The agency is just beginning to discuss technology that would continue to “learn” as it processes thousands of diagnostic scans. Forget scraping the NYT website, the data that would be used for this would be hugely invasive.
Humans go through years of rigorous learning before being granted diagnostic authority – shall we hold AI to the same standard? And if so, would it still take years, or because of its learning speed, would we hold the technology to be fully qualified after a much shorter time?
If so, what price doctors any more?
FDA rules and approvals on AI in medicine
The FDA’s existing rules encourage developers to focus on one problem at a time – a heart murmur or brain aneurysm – whereas AI in medicine in Europe scans for a range of problems.
Because the American healthcare system is for-profit, the agency’s reach is limited to approving products that are for sale – it has no authority over programs that health systems build and use internally.
Large healthcare systems like Stanford, the Mayo Clinic and Duke – as well as, and this might be scariest, health insurers – can build their own AI tools affecting care and coverage decisions for thousands of patients, with little to no government oversight.
Doctors are raising questions as they try to deploy the roughly 350 FDA-approved software tools. How was the program built? How many people was it tested on? Is it likely to identify something a typical doctor might miss?
The lack of publicly available information is causing doctors to hang back, wary that technology that sounds exciting can lead patients down the road to more biopsies, higher medical bills and toxic drugs, without significantly improving healthcare.
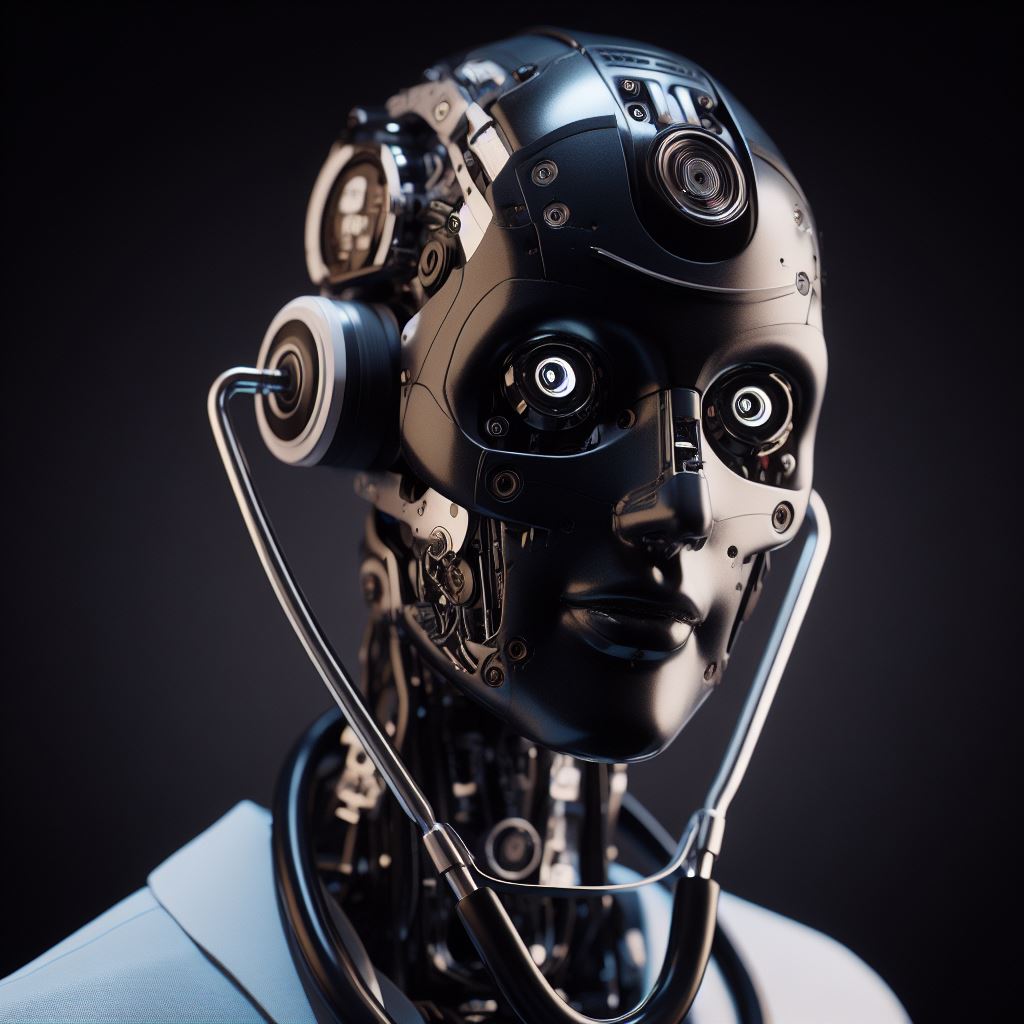
Don’t worry, it’s listening. Or maybe do… (AI-generated image).
So, AI in medicine comes at higher price for the same level of healthcare. Or even, if you take into account the human impact of doctors on the provision of healthcare, potentially worse care for more money.
Dr. Eric Topol, author of a book on AI in medicine, is optimistic about the technology’s potential. But he said the FDA had fumbled by allowing AI developers to keep their “secret sauce” under wraps and failing to require careful studies to assess any meaningful benefits.
“You have to have really compelling, great data to change medical practice and to exude confidence that this is the way to go,” said Topol, executive vice president of Scripps Research in San Diego. Instead, he added, the FDA has allowed “shortcuts.”
But then, if not for the smoke and mirrors, how would doctors like Topol have such unwavering optimism about AI’s potential?
Too much, too soon, with too little testing?
Large studies are beginning to be published, according to Topol: one found the benefits of using AI to detect breast cancer, while the other highlighted flaws in an app meant to identify skin cancer.
AI has made a major inroad into radiology; some estimate that around 30% of radiologists are using the technology already. Simple tools that might sharpen an image are an easy sell, but higher risk ones like those selecting whose brain scans should be given priority are worrying – and not just for doctors.
After the “godfather of AI” Geoffrey Hinton said, in 2016, that AI might replace radiologists altogether, the profession didn’t necessarily welcome the technology.
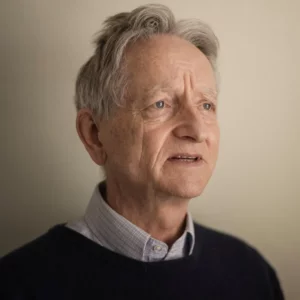
Geoffrey Hinton, image via the Guardian.
Aware of potential flaws in immature models, Dr. Nina Kottler is leading a multiyear, multimillion-dollar effort to vet AI programs. She is the chief medical officer for clinical AI at Radiology Partners, a Los Angeles-based practice that reads roughly 50 million scans annually for around 3,200 hospitals, free-standing emergency rooms and imaging centers in the United States.
Kotler said she began evaluating approved AI programs by quizzing their developers, then tested some to see which of them missed relatively obvious problems – or pinpointed subtle ones.
She rejected one approved program that didn’t detect lung abnormalities beyond the cases her radiologists found and missed some obvious ones.
Another program that scanned images of the head for aneurysms, a potentially life-threatening condition, proved impressive, she said. It detected around 24% more cases than radiologists had identified. More people with an apparent brain aneurysm received follow-up care, including a 47-year-old with a bulging blood vessel in an unexpected corner of the brain.
“Apparent” aneurysms is a key word there, though: the technology flagged many false positives.
There are, absolutely, success stories in the use of AI in medicine. In August, Dr. Roy Fagan realized he was struggling to communicate with a patient. Suspecting a stroke, he rushed to a hospital in rural North Carolina for a CT scan.
The image went to Greensboro Radiology, a Radiology Partners practice, where it set off an alert in a stroke-triage AI program. A radiologist didn’t have to sift through cases before Fagan’s or click through more than 1,000 image slices; the one spotting the brain clot popped up immediately.
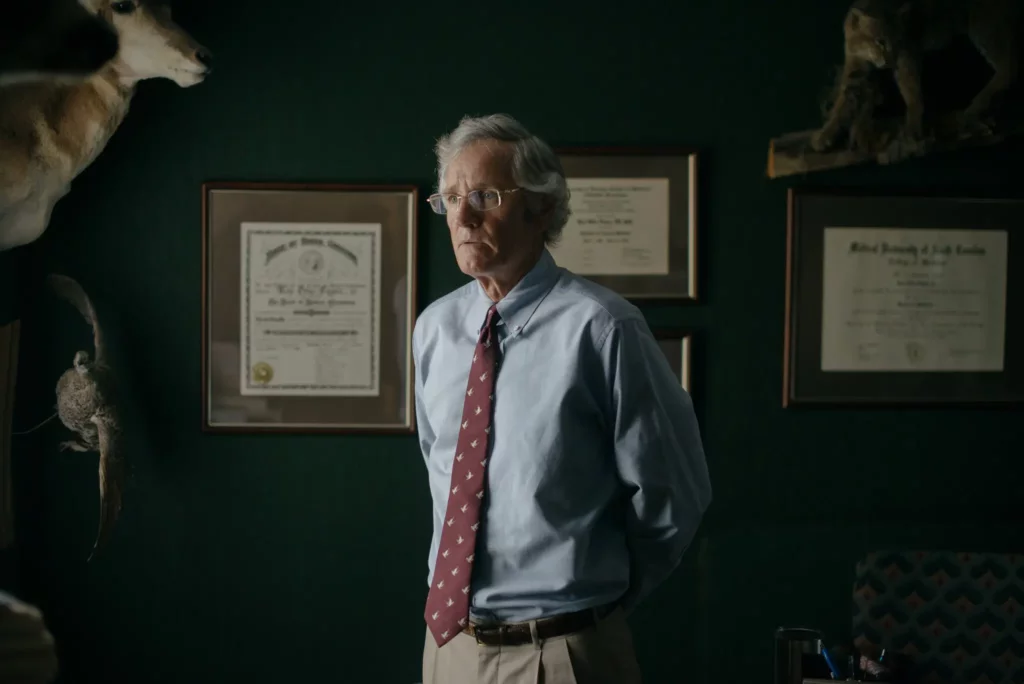
Dr. Roy Fagan may well have had his life saved by AI in medicine. Credit: Jesse Barber for The New York Times.
Fagan could then be transferred to a larger hospital and receive medical intervention. After waking up feeling normal, Fagan was impressed with the program. He said, “it’s a real advancement to have it here now.”
There are also the less inspiring examples of AI in medicine. A program that analyzed health costs as a proxy to predict medical needs ended up depriving treatment to black patients who were just as sick as white ones. The cost data turned out to be a bad stand-in for illness, a study in the journal Science found, since less money is typically spent on black patients.
But a computer program needs a proxy for illness, given that it can’t gauge something so elusive as pain.
The program hadn’t been vetted by the FDA, but when, having witnessed an unapproved system fail, doctors looked to agency approval records for reassurance, they found little.
One research team looking at AI programs for critically ill patients found evidence of real-world use “completely absent” or based on computer models.
The pressures of procedure
In 2021, a study of FDA-approved programs found that of 118 AI tools, only one described the geographic and racial breakdown of the patients the program was trained on. Most of the programs were tested on 500 or fewer cases — not enough, the study concluded, to justify deploying them widely.
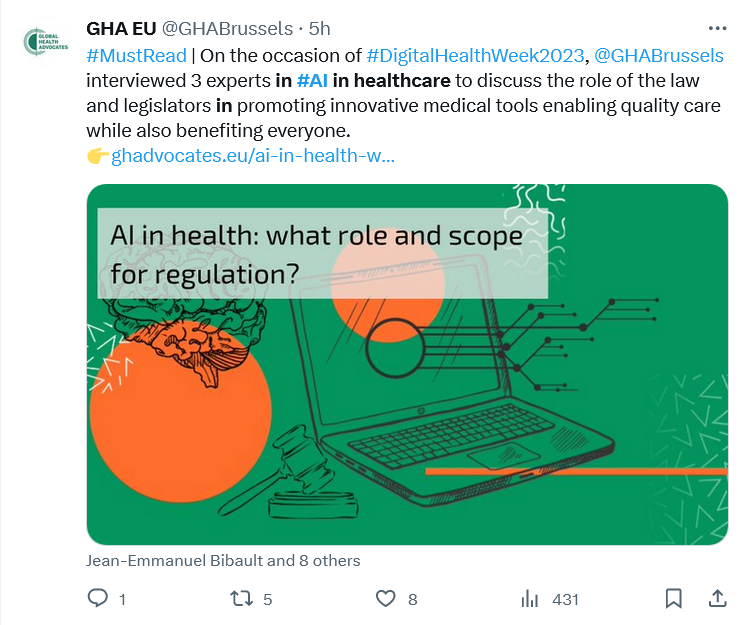
Is AI in medicine safe enough for widespread use?
James McKinney, a spokesperson for the FDA, said the agency’s staff members review thousands of pages before clearing AI programs, but did acknowledge that the publicly released summaries may be written by the software makers.
Those are not “intended for the purpose of making purchasing decisions,” he said, adding that more detailed information is provided on product labels, which are not readily accessible to the public.
While AI is still, relatively speaking, in its early development, it feels premature to expand its use in medicine beyond administrative tasks. Even then, the issue of privacy might be enough to cause a draw back from AI in medicine.
That being said, AI is where the money is, and a private healthcare system tends to follow the cashflow. And when patients are by necessity consumers of product, their importance in the equation of system development and sale is automatically, and perhaps dangerously reduced.