4 terrifying dangers lurking in AI
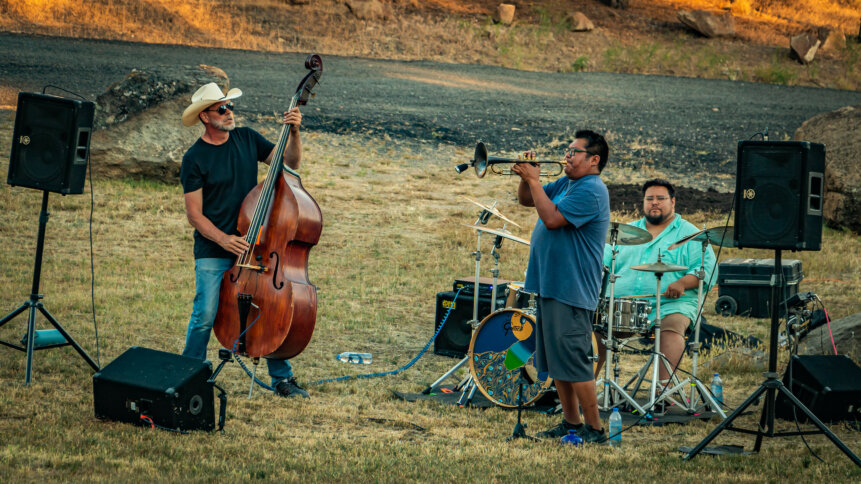
Now that AI is well and truly embedded into the collective consciousness, it’s time that we, as technologists, parse some of the real and imagined ‘dangers’ lurking in the technology.
For the purposes of argument, let’s first assume that AI, in the common parlance, is equated with machine learning (ML), and in the public perception, at least, LLMs (large language models).
To understand AI, we must have at least a cursory grasp of how the technology works. Many commentators feel fit to pass judgment on the implications of AI without actually understanding the basics of what goes on under the hood. In that, there’s nothing wrong per se: plenty of professional car enthusiasts out there, for instance, wouldn’t know their crankshaft from their big end. But a grasp of the processes involved in producing a recognizable AI, specifically, an LLM, helps explain how and why certain dangers exist.
Machine learning models of any type need a body of data from which to learn. A large quantity of data is generally considered better than a small one, and clean data is usually preferred. Clean data exhibits as few anomalies as possible in its structure (so all international ZIP codes should be made to follow the same format, for example) and in its content, too. Bodies of information fed to an AI that state too often that the world is flat will influence the model’s perceptions of what shape the world is. This example neatly brings us to our first deadly danger:
AI is biased
It’s accepted wisdom that any body of data will contain outliers – snippets of information that are well off the beaten track compared to their peers. Among a list of popular religions, for example, there will be one or two latter-day wits that claim to follow the ways of the Jedi Knights. A smart AI algorithm can cope with outliers and not adjust its comprehension to an inappropriate degree. However, if the body of information given for learning is inherently biased, in the main, then the “taught machine” exhibits the same attitude.
YOU MIGHT LIKE

A Github Copilot thought experiment
Large parts of the internet, for example, are dominated by young, Western men interested in computing. Sampling data from there would lead any learning algorithm to believe there are few women, few old people, and few people with so little disposable income they couldn’t afford the latest technology. In the context of the learning corpus, that may be true. In a wider context, not so.
Therefore, any learned picture of the world drawn from the internet reflects the inherent bias of the personalities present on the internet.
Inaccuracy
Machine learning algorithms will harvest data that presents a biased picture, and extrapolated conclusions requested by end-users querying Bing’s AI, for example, will reflect that. It may present conclusions of the ‘fact’ that young American males of color have strong criminal tendencies. That’s not because of any truth in that finding; it’s because a political system has incarcerated that demographic to an extraordinary degree.
Large language models are created by a complicated, statistically variable word-guessing game. OpenAI’s ChatGPT, for example, has learned to communicate by compiling sentences from lists of words, one after another, based on what the next word is fairly likely to be.
This process can lead to AI “dreams,” beloved by the mainstream press. Once anomalies creep into the real-time guesswork of what word comes next, errors that form surreal imagery compound, creating streams of consciousness that amuse and confound in equal measure.
Copyright or license infringement
Creative works or everyday internet postings are released under some degree of stricture, deliberately by the author or from those given by a proxy. The contents of Twitter (or X), for example, are owned by the company running that platform. Pictures taken from a high school reunion on Facebook (Meta) are owned by Mark Zuckerberg. And computer code written under a deliberately chosen license (the GPL, for example) has similarly to be reused or represented in a particular way.
When MLs are presented with raw data, however, it’s not clear whether or not any licensing strictures are observed. Does OpenAI grab copyright material to learn its language? Does Bing Image Creator take copyright imagery to learn how to paint? And if the greedy silicon digestive systems then spout, in part or whole, material that was released restrictively, where does the end-user stand in the eyes of the law?
Like the legal complications of liability in the event of a crashed autonomous vehicle, the new paradigm is unexplored territory, morally and legally. Authors, artists, and programmers may protest their work is put to uses it was never designed for, but the internet age’s adage of ‘be careful what you post’ is especially relevant now.
Even if creators somehow flag their output as ‘not to be used by learning models’, will the large operators of those models respect their choices? Like the “do not follow” entries in a website’s robots.txt file; it’s debatable whether any individual’s wishes are respected.
YOU MIGHT LIKE
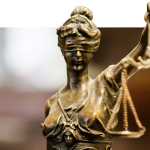
Responsible AI takes more than good intentions
Mediocrity
From the early days of computing, data’s veracity was always doubtable. GIGO (garbage in, garbage out) remains a cornerstone of data analysis. In 2023, media companies began to use LLMs as content producers for various purposes: item descriptions in large online stores, reports on financial markets, and articles that contain perfect keyword densities to produce optimized SERP (search engine results page) placement.
And because the LLMs continue to snapshot the internet as new learning corpora, there is a significant danger of a spiral of self-propagation. Artificial intelligences will begin creating new generations of learned ‘facts’ that were themselves produced by AIs.
Ask a large language model to explain, for example, mental health law in Canada. The results will be coherent and comprise readable paragraphs and use bullet-point summaries of key information. The choice of bullet points comes not from the importance of any bullet-ed statement but from the fact that years of SEO practise have stipulated that bullet point lists are a good way to create web content that will rank well on Google.
When that information is copied & pasted into new articles and then absorbed in time by LLM spiders crawling the web, the decision to use bullet points becomes reinforced. The information in each snappy highlighted sentence gains extra emphasis – after all, to all intents and purposes, the author felt fit to highlight their statement in this way. It’s easy to see the dilution of importance by repetition, as evolving LLM models merely repeat and refine emphasis that was never particularly justified.
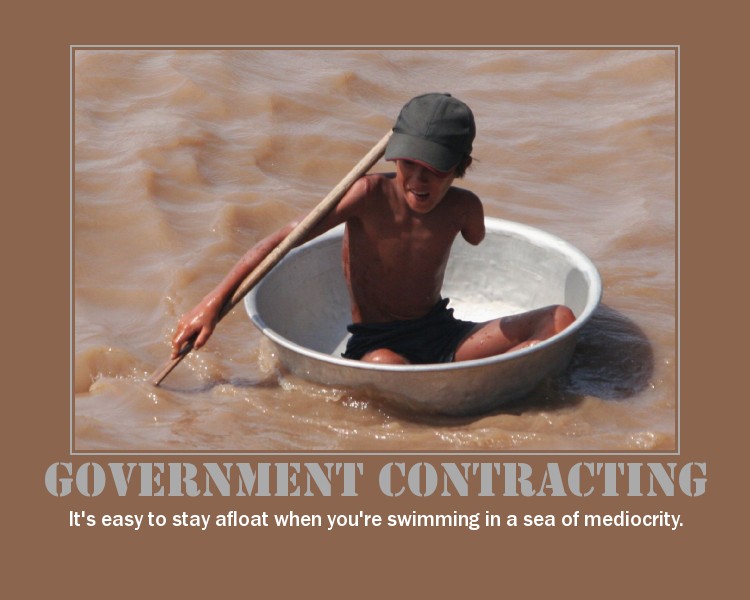
“DEMOTIVATIONAL POSTER: Government Contracting – It’s easy to stay afloat when you’re swimming in a sea of mediocrity.” by Claire CJS is licensed under CC BY-NC-SA 2.0.
Over the years, average humans will produce average content consumed and averaged out by LLMs, producing even less remarkable content for the next generation of OpenAI-like companies to consume. Mediocrity becomes the norm.
Brilliant art, amazing writing, and earth-changing computer code can be produced by talented people, only to be subsumed in a morass of “meh” and regarded only as an outlier and disregarded by algorithms trained to ignore or at least tone down extraordinary content. There’s no consideration of value, merely distance from the average as a measure of worth.
Perhaps in that, there is a gleam of hope. If machine learning’s output is merely passing fair, genuine creativity will surely stand out. Until some very clever people quantify the muse and write algorithms that easily out-create the human creators.