Speech recognition is booming, but only selectively
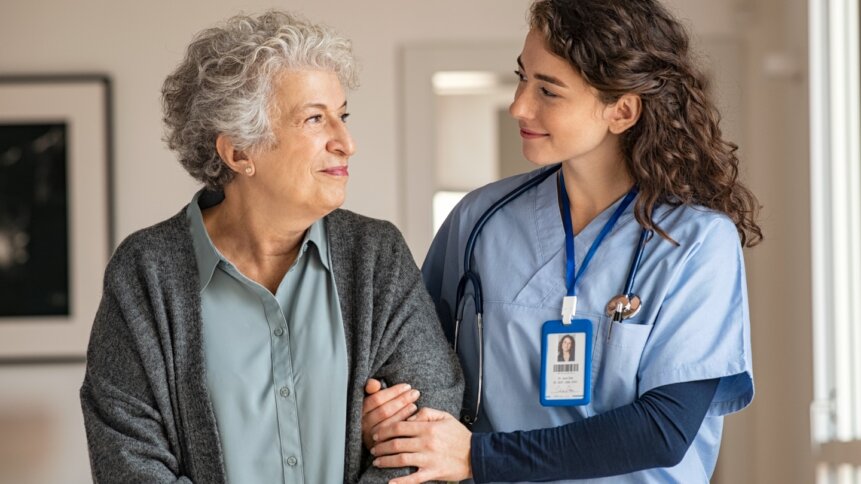
Less than a couple of decades ago, the immediate prospects for speech recognition weren’t great. Digital dictation services were in their infancy – costing thousands of dollars to purchase, requiring hours of user training, and delivering a customer experience that was, let’s say, variable. But fast forward to the present day and that’s all changed – big time.
Just last year, Nuance – a pioneer in the market with its ‘Dragon Dictate’ product back in the 1990’s, a value proposition that was based on speeding up the transfer of spoken words onto the digitally rendered page – was purchased by Microsoft for almost $20 billion. And digging into the details, you can see why. Over time, Nuance has found a very appreciative audience for its voice recognition products – not typists, but doctors. And, taking healthcare as our first example, the savings really add up. Nuance posts that users of its ‘Dragon Medical One’ product – a document companion app for clinicians – typically save up to two hours per person per shift, allowing doctors to spend more time with patients and cutting the duration of document-related tasks in half.
Two-way conversation
According to the US firm, its speech-powered solution is five times faster than typing and is used by over half a million clinicians. And it’s not just about automated notetaking, medics can quiz patient records verbally to identify medication needs, for example, and then – again, using voice – place orders and set reminders for calls. Nuance claims that its recognition engine provides 99% accuracy, which goes a long way to explaining its success. And this reliability has opened the door to uses elsewhere such as in the financial sector.
In banking, the company has teamed up with the UK’s Nat West group, a provider of financial services to 19 million customers across 12 brands, to help reduce fraud. Thanks to voice biometric technology – derived from speech recognition – the group has been alerted to more than 23,000 potential incidents of fraud. And the savings add up to a return on investment of more than 300% – which makes for an easy conversation for managers with their purchasing departments.
Being able to trawl speech data provides insights beyond security, and management consultants McKinsey & Company offer insights on this front. Applying natural language processing techniques to call centre data can help with better business forecasting by tracking which calls fall into which category – for example, bookings, cancellations or modifications to offers. McKinsey’s analysts estimate that the use of automated speech analysis tools can deliver savings in the region of 20-30% for operators, compared with manual call sampling, which – rather than scouring all customer interactions – likely only capture details on 2%, or less, of the calls received. They make a great point too about recording quality, noting that poor audio doesn’t just affect customers and operators – it ramps up the number of errors made by speech recognition services. Another reason to invest in good quality headsets for your staff (‘crystal clear comms’ was one of TechHQ’s 5 enterprise IT projects to refresh strategy in 2022).
The insights and time savings provided by speech recognition have closed the deal on whether customers should invest in solutions – at least for firms operating in English-speaking markets. Studying data sets of the size required to develop a slick speech recognition system is expensive and represents a big financial commitment. It’s meant that developers have drilled down on more widely spoken languages such as English and Mandarin – powering some impressive, albeit language-specific, gains in performance. Even five years ago, researchers based at Stanford University and the University of Washington working with experts at Baidu Research, found that ‘speech was 2.9x faster than typing for both English and Mandarin Chinese’ – for general purpose text entry using smartphones.
Unequal language resources
Writing in VentureBeat, Ricardo Baeza-Yates –a professor at Northeastern University’s Institute for Experiential AI in the US – comments that unequal language resources are one of the major limitations of language models in general. Considering text and using Wikipedia as a convenient data set, Baeza-Yates notes that out of around 7,100 languages currently spoken, only 312 have active entries on the site – which is one of the most popular destinations on the web. The fraction represents just 4.4% of all languages and he adds that of those 312 active entries, only 34 are associated with more than one million pages. Language models go hand-in-hand with speech recognition as they assist in the back end, helping to decode – together with acoustic references – the most likely candidate for features that would otherwise confuse systems.
There are some workarounds to support additional languages, as Julien Salinas – Chief Technology Officer at NLP Cloud (a French tech firm providing API’s to help businesses to capitalize on advances in machine language learning) – points out. One of the hacks is to use translation, which has support for many languages, even obscure and endangered ones (Google’s Woolaroo recognises objects in photos and translates them into indigenous languages).