Malware protection: deep learning leads cybersecurity charge
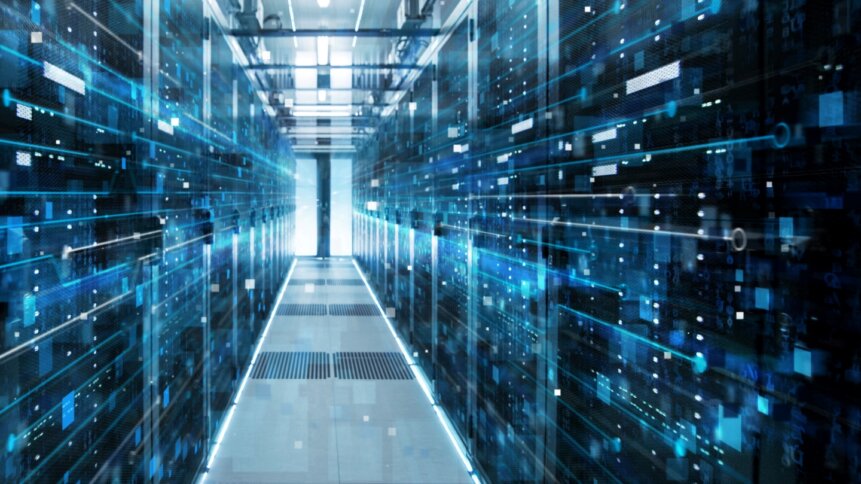
The power of deep learning to play chess, go, and answer Jeopardy questions (not to mention Agent57 outshining human players on the classic Atari computer game, Pitfall) is impressive. Such achievements represent milestones in artificial intelligence (AI), but they are not applications that most companies can benefit from directly day-to-day. The use of deep learning to stop malware in its tracks in less than 20 milliseconds, on the other hand, definitely ticks the practical applications box and has got businesses very interested indeed.
Whistle-stop tour
Malware protection has come a long way from its early signature-based anti-virus (AV) origins. Heuristic analysis came next, as providers looked to build a first step in defending against unknown attacks. But the behavior patterns weren’t flawless and the penalty for trying to spot malware that had yet to be added to virus definition files was false positives. This led companies to explore machine learning (ML) methods to tease out characteristics that separated dangerous payloads from similarly looking, but harmless, software. However, there were still drawbacks.
The challenge for the firms deploying ML algorithms, is the level of human intervention required to prepare the training data – a step known as ‘feature engineering’. The reward is a more accurate model, but time constraints typically mean that the tuned algorithm is only based on a small fraction of available data. In contrast, deep learning gets to work on 100% of available raw data – testing and re-testing itself to build a multi-layered statistical model that, over time, is able to infer a vast amount of detail from its inputs.
So far, so good, but why didn’t the cybersecurity world just jump straight to the deep learning part and be done with the rest? The big reasons are time and money. Deep learning, as IBMs Jeopardy-winning project demonstrates, requires considerable resources. Advances in processing hardware are helping to accelerate the long trawl that’s required to digest the vast data sets that are necessary to access deep learning’s rewards. And even today such endeavors require significant amounts of investment, but the jump in performance that’s obtained has grabbed the attention of investors.
Putting AI to the test
In the summer of 2021, cybersecurity firm Deep Instinct raised a further $67 million (taking the total investment raised to around $240 million) to further develop its deep learning based threat prevention platform. Tellingly, one of the firm’s early backers was Nvidia – a designer of graphics processing units (GPUs) that have proven to be well-suited to AI processing, including deep learning.
Recently, in 2022, Deep Instinct invited white hat security experts Unit 221B (and yes, the information security group is a big fan of Sherlock Holmes – if you were wondering about the name) to put its Endpoint Protection Platform (EPP) to the test. And the results were a big thumbs up for the power of deep learning to take cybersecurity to new heights.
“Deep learning is much better at telling what’s good and what’s bad,” Justin Vaughan-Brown, who joined Deep Insight’s VP team ahead of the Unit 221B assessment, told TechHQ. “And if you know what’s good, you can let it though.” Vaughan-Brown points out that in the absence of deep learning solutions SecOps teams can spend a large proportion of their time dealing with false positives. And for businesses, this impacts the bottom line. Not just in wasted time for cybersecurity and IT staff, but due to the delays in releasing business-critical documents that turn out to have been held up unnecessarily.
Deep Instinct reports that its platform has a false positive rate of less than 0.1%. The figure is so low that Vaughan-Brown has known of new customers double-checking whether their system is running correctly (which it is) as they can’t believe the reduction in erroneous alerts. The predictive powers of the deep learning engine benefit users in other ways too. And one of the biggest wins is being able to detect unknown attacks.
During the third-party study, where Unit 221B analysts targeted test machines featuring Deep Instinct’s end-point agent with a battery of digital assaults, the EPP was successful in preventing 437 unknown malware attacks with 100% accuracy. And for all tests conducted across the engagement, no false positives were identified. In fact, Deep Instinct is so confident in the ability of deep learning to piece together the characteristics of a new attack, that updates are required only infrequently – making its tool as capable offline as it is online.
Performance guarantee
The firm’s confidence extends to stopping ransomware too, and this is where the speed of response comes to the fore. Fast-acting, the platform can catch malware before it executes. “Prevention is better than clean up,” emphasizes Vaughan-Brown. And while there may be mixed views on ransomware insurance in some quarters, Deep Instinct backs its solution with a $3 million warranty facilitated by German insurance experts Munich Re.
To prepare for the deal, Munich Re’s actuaries spent a week at Deep Instinct’s facility in Tel Aviv carrying out extensive due diligence on the cybersecurity firm’s technology. And Deep Instinct is one of a growing number of partners in the insurance firm’s aiSure program, which provides a guarantee of the performance of AI solutions.