AI water footprint suggests that large language models are thirsty
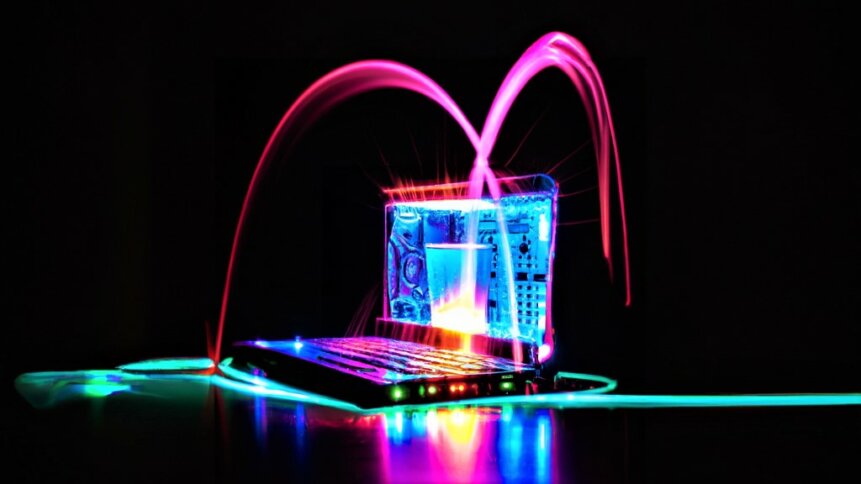
Getting your Trinity Audio player ready...
|
There’s much to celebrate about the success of conversational AI tools such as OpenAI’s ChatGPT, which is rapidly automating the business landscape thanks to a raft of enterprise integrations. But creating large language models (LLMs), such as GPT-3 and its successor GPT-4, turns out to be thirsty work. And attention has turned to the AI water footprint accompanying this game-changing trend.
At the heart of LLMs are billions of parameters tuned using vast amounts of data scraped from the web. The resulting algorithms have proven to be spookily effective at responding to user text prompts, can speak multiple languages, and understand code. But this performance comes at a cost. LLMs can take months to train, even using supercomputers that have been custom-built for the task. And the heat generated by all of that compute has to be managed to maintain ideal operating conditions.
Modern data center heat management solutions include closed-loop liquid cooling that passes warm water exiting the server rooms through a chiller heat exchanger and back to the racks of processors. Closed loops are good news for liquid consumption, but this is only half of the thermal story. On the other side of that heat exchange process are typically a number of evaporative cooling towers, which sit outside the cloud computing facility and have to be topped up with on-site water.
AI water footprint calculator
“Despite recent advances in cooling solutions, cooling towers are dominantly the most common cooling solution for warehouse-scale data centers, even for some leading companies such as Google and Microsoft, and consume a huge amount of water,” write Shaolei Ren and colleagues in a new study estimating the fine-grained AI water footprint of LLMs such as GPT-3 and other generative AI algorithms.
Operators are taking steps to reduce data center water consumption – for example, by leveraging outside cold air when temperatures permit, using non-potable water, and reusing warm water for heating nearby offices and residences. But these solutions aren’t sufficiently widespread to have made the AI water footprint problem go away.
In fact, while much has been made of the carbon footprint associated with the use of advanced AI – some analysts have concluded that the environmental impact of training LLMs could be comparable to hundreds of aviation passengers taking multiple long-haul flights – the enormous water footprint of AI models has remained under the radar. In their study, Ren and his team – based at the University of California, Riverside, and the University of Texas at Arlington – estimate that training GPT-3 could have consumed as much water as required to produce 370 BMW cars or 320 Tesla electric vehicles. What’s more, the group believes that water consumption would have been tripled if training had been conducted using facilities that weren’t state-of-the-art. And the concerns don’t stop there.
Model inference – the process by which a trained generative AI algorithm returns an output based on an input prompt – can be resource intensive too. “ChatGPT needs to ‘drink’ a 500 ml bottle of water for a simple conversation of roughly 20-50 questions and answers, depending on when and where ChatGPT is deployed,” comment the US-based researchers in their paper, which lists the assumptions feeding into their calculation.
Water carbon conflict
Data center operators will be quick to point to the dramatic growth in the use of wind and solar and the contribution that green technology makes to shrinking cloud computing’s carbon footprint. But optimizing water usage can be at odds with peak clean energy. For example, maximum solar generation will occur during high-temperature hours of the day, when cooling systems will be thirstiest. And conversely, during the night, when outside temperatures will be lower and more favorable for thermally efficient data center operations, solar has packed up and gone to bed.
The researchers highlight that ‘when’ and ‘where’ matter in determining the environmental costs of training large AI models. And they have some interesting proposals for addressing the AI water footprint of ChatGPT and related LLM projects. One idea is to adopt federated learning strategies – in other words, encourage multiple users to collaborate on training AI models using local devices, which don’t consume on-site water. Information from electricity providers could also be integrated into the process, dynamically distributing the training load according to the availability of clean energy.
Developments in energy storage will help here too. For example, hydroelectric facilities and large battery arrays could buffer solar and wind energy when its readily available and time-shift the deployment of clean electricity to coincide with water-efficient thermal management opportunities. And there are signs that the tech industry is beginning to consider the positive impact of giving users the option to schedule device charging and operating system updates – based on recent updates made by Apple and Microsoft, to give a couple of examples.
As is often the case, transparency is key. And just as it’s good practice to accompany trained machine learning models with so-called model cards that inform users on the data sources involved and warn of potential biases, it could also to be helpful to acknowledge when and where training took place to address AI water footprint concerns.