AI parenting, bias and diversity
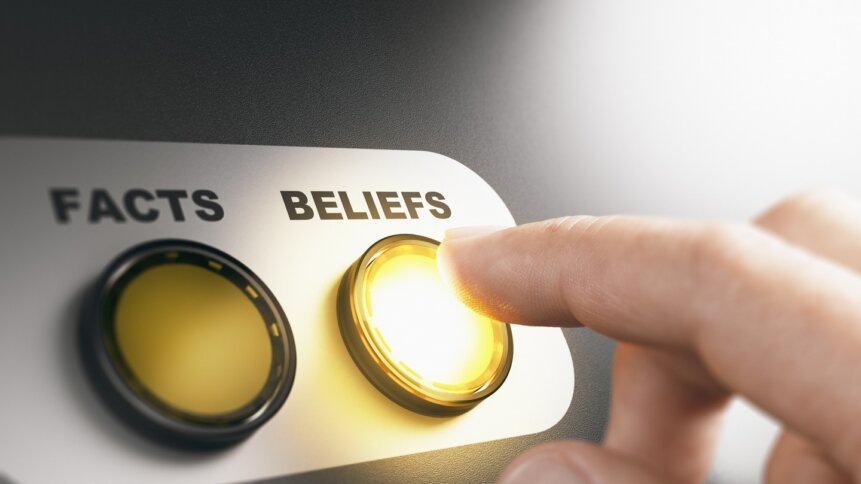
• AI parenting is the process of training your generative AI to do the right things.
• The opportunity for bias to creep into AI is enormous, because it will tend to reflect the biases of the people who build it and the society in which it functions.
• You have to actually put a lot of effort into diversifying your AI from start to finish.
READ NEXT

The art of AI parenting
Generative AI is already a must-have technology for many organizations, and it’s still less than a year since ChatGPT exploded onto the market and made businesses and regulators both spit out their Wheaties. But if we’re going to evolve into a society where AI underpins everything we do, we need to ensure that our AI parenting is up to scratch.
Why? In Part 1 of this article, we sat down with Dan O’Connell, chief AI and strategy officer, and Jim Palmer, VP of AI engineering, at Dialpad, a cloud communication company that has called for such rigorous AI parenting, to try and find out.
Dan and Jim explained that, just as with any child, there was a need to steer, to guide, to check development and to course correct, so that the end result is something you can take out in polite modern society without it talking confident nonsense or actively insensitive idiocy [Insert your own political joke here].
That’s what AI parenting is – the process of making sure your AI steers clear of bad influences like the real world we’ve built or the internet that mirrors it, and instead delivers useful insights on your data, reliably over time.
AI parenting – bringing up baby.
What became clear while talking to Dan and Jim was that firstly, speech recognition across a potentially broad spectrum of languages, dialects and linguistic quirks is crucial to getting your AI parenting right – because getting it wrong means your AI might miss important social or cultural contexts, which would make it as fallible as a human being.
We wondered how you ensured the sincerity of your AI parenting when potentially, everybody was using either the wild west that is the ungoverned internet to try and parent their AI, or a limited funnel of oven-ready third-party software to deliver that AI parenting.
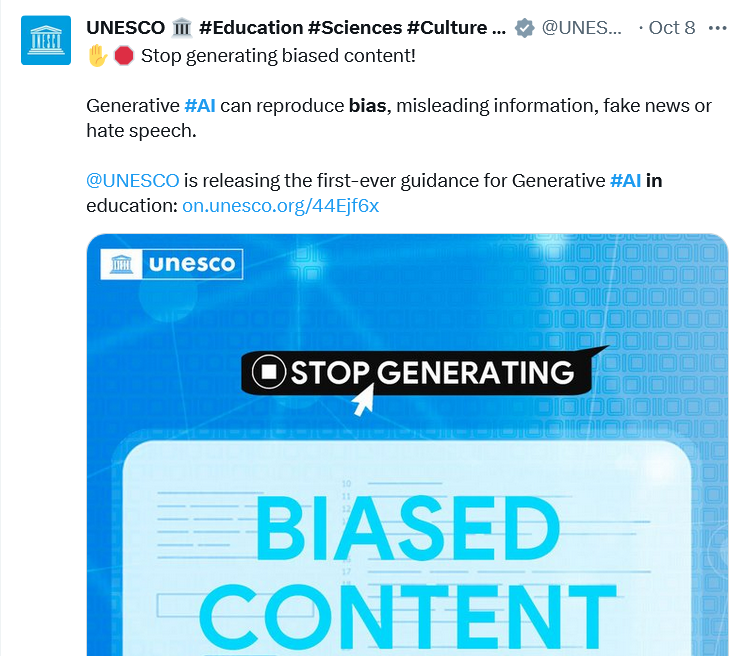
AI bias – it’s a real issue.
DO’C:
Ah, well, there we’re a little… unusual. We may be the only provider in the game that owns their entire AI stack. So we do all of our own transcription without using third-parties. We do all of our own NLP modeling, so if we wanted to identify things like a sentiment, named entity recognition, identify action items, anything of that nature, we have our own semantic search that can get us there. That allows us to build recommendation engines, suggested answers to questions, and then we do our own model work from there.
That means we can train the large language model by transcribing all of these conversations happening on the Dialpad platform. That’s opted-in data, obviously, stripped of any personal identifiable information and anonymized. And that allows us to safely build these additional features that provide value.
THQ:
So the issue we raised… remains an issue for the wider world, but you guys build from the nuts and bolts up.
DO’C:
Exactly.
AI parenting to eliminate bias.
THQ:
Okay. Let’s talk about bias for a minute. We’ve spoken to lots of DE&I people, and lots of women in tech, and they’re clear on this – unless we get our AI parenting spot on, we risk simply training our AI models to be as skewed as our society has traditionally been. So for AI to be the aspirational technology it could be, and for it to have a chance of genuinely improving the world long-term, we have to kill the bias we might otherwise introduce to the system, don’t we?
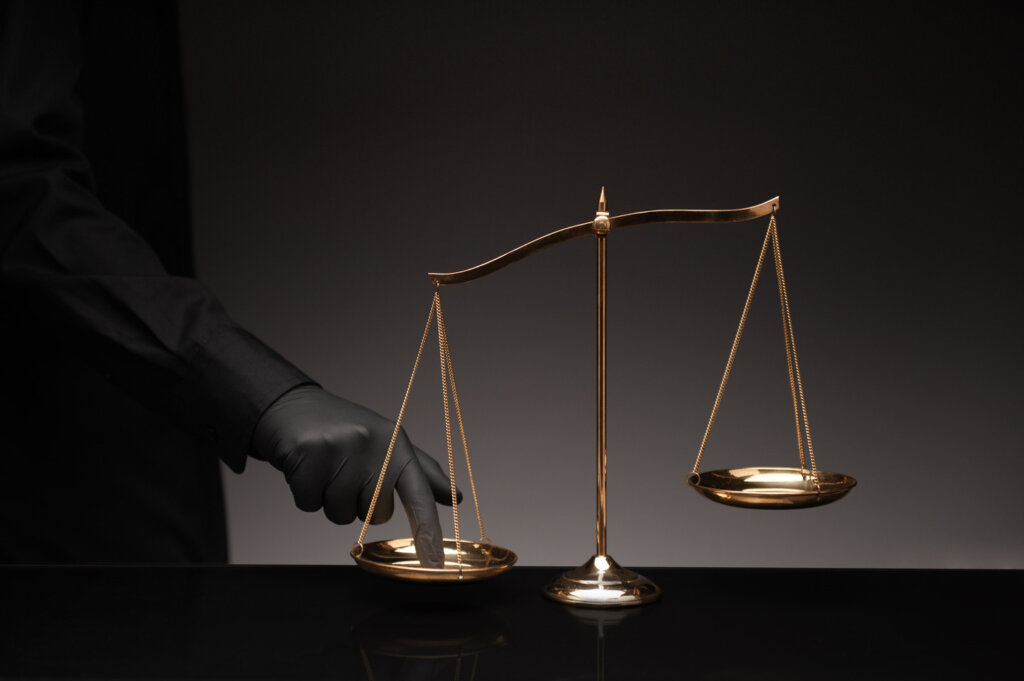
AI parenting in an inherently biased society is…tricky.
DO’C:
Yeah. It’s important thing that we recognized these things early on, so we actually have guiding principles for AI parenting among our teams. We need to make sure that we’re building features that enhance the experience the AI delivers. AI is supposed to help you get promoted, not to replace you.
To make sure it does that, we have to get into how we ethically build an AI system that is useful, transparent, and minimizes the bias that’s fed into it. We have a team that that is focused specifically on that, and on delivering on these guiding principles. One important question in the whole AI parenting process is how we test for these biases. That’s a very, very real problem that shows up.
JP:
This has been an initiative for us. We have a team, we have a panel, we have leadership throughout our AI stack participating in the entirety of our engineering product design, and across the entire organization. It’s been there from the get-go.
THQ:
OK… but what does it actually do?
JP:
It all comes down to every single training exercise throughout the model development, whether it’s just a very lightweight initial test, or the assessment of how much data we have, or what data we have access to. This is a really important part – we have an in-house data and annotation team, a full-blown data team. They’re not really the classic data analysts, these are linguists who also adhere to our core AI principles.
We also have a team of scientists, so they’ve taken that sort of scientific oath in terms of being responsible with this data. So we’re not just using our training data to test with, all of those things are deeply rooted in every single one of the scientists that we have on our team.
Diversity in AI parenting teams.
What it all comes down to is trying to solve this the best way we can in terms of diversity from the get-go. Where can we get the data? How are we doing the breakdown?
I see a few companies being responsible with their AI parenting on diversity, but to discover that, you have to ask how they’re representing the data that they’re using, and how they’re classifying the data that’s going into the training, and how public they’re being about that.
We have a lot of data on this for every project we do. We have internal data cards that are very raw and rough, but we know what our geographical distribution is, we know some of the classifications to help us understand the diversity of our data going in, and that goes for our speech recognition, our training data for all of our NLP training, and for every model that we’ve done.
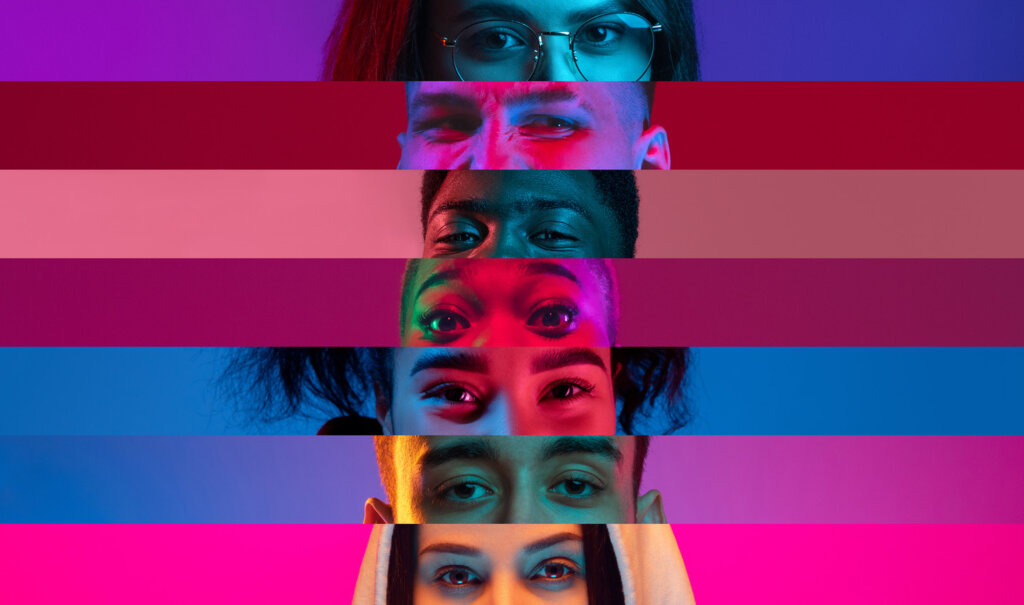
Engineering in diversity.
DO’C:
And the other point of course is that we have a diverse team. So it’s not only diversity in the dataset, but we have diversity in the thought processes and diversity in the teams that are working on the projects.
If everyone looks like us, a couple of white guys, then obviously, they’re gonna come with their own biases and upbringings, and that’s going to unnecessarily narrow the funnel of experience going into the work. So we’ve got a very diverse team, and those guiding principles, and the data insights, to try and almost engineer out the biases that the work might otherwise have.
THQ:
It’s fair to say that two years, five years down the line, what acceptable diversity and inclusion standards look like will be a world away from where we’re at right now.
DO’C:
That’s where I think we have an advantage. We’ve been positioning our AI strategy around that last untapped resource, those business conversations, the phone conversations.
We’re not going to say that we have an AI reckoning in that sense. But getting more diversity for the texts and the audio is going to be a long-running problem for all of AI. So I’m happy that there are a lot of companies, academic institutions, and everything in between that’s really taking that seriously.
But where we have an advantage is that we get a lot of that diversity for free in our data from our customers. It’s our responsibility to do the right thing with that data.
The danger of bias in generative AI.
In Part 3 of this article, we’ll take AI parenting down to the nuts and bolts of how you do the job.