IBM to use in-house AI chip to reduce costs of Watsonx
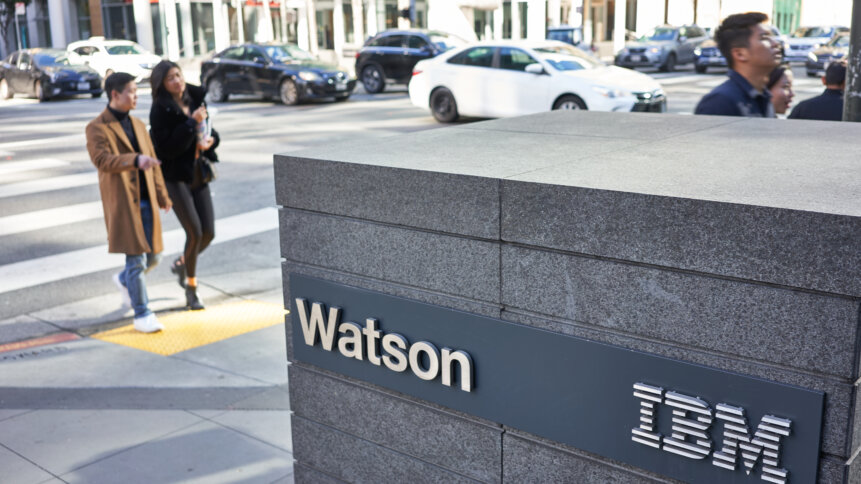
• IBM to use its own in-house AI chip for Watsonx.
• The company hopes to tap a market in the age of generative AI.
• Using an in-house AI chip could save IBM crucial expenditure on the project.
It’s been a while since IBM has actively touted Watson — the tech giant’s early splash in artificial intelligence, that never amounted to a profitable offering.
Today, Watson has given way to WatsonX, IBM’s new enterprise-ready AI and data platform, indicating that the Big Blue is back in the game, this time trying to ride the latest boom in AI. But to avoid past mistakes in cost management, IBM plans to utilize its own in-house AI chip.
IBM is billing the platform, Watsonx, as a development studio for companies to “train, tune and deploy” machine-learning models. The platform includes a feature for AI-generated code, an AI governance toolkit, and a library of thousands of large-scale AI models trained on language, geospatial data, IT events, and code, according to a release.
The tech giant first unveiled it in May this year, and on July 11, this week, IBM revealed that it had been shaped by more than 150 users across industries participating in its beta and tech preview programs.
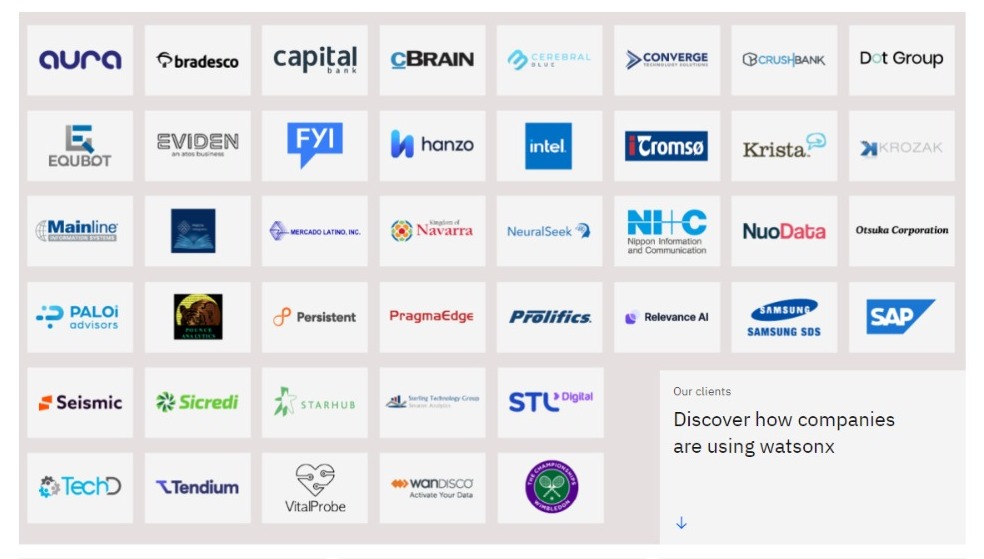
Clients using Watsonx. Source: IBM
“Previewed at IBM THINK in May, Watsonx comprises three products to help organizations accelerate and scale AI – the watsonx.ai studio for new foundation models, generative AI, and machine learning; the watsonx.data fit-for-purpose data store, built on an open lakehouse architecture; and the watsonx.governance toolkit to help enable AI workflows to be built with responsibility, transparency, and explainability (coming later this year),” IBM said in a statement.
Simply put, Watsonx allows clients and partners to specialize and deploy models for various enterprise use cases or build their own. “The models, according to the tech giant, are pre-trained to support a range of Natural Language Processing (NLP)-type tasks including question-answering, content generation and summarization, text classification, and extraction.”
A déjà vu?
In February 2011, the world was introduced to Watson, IBM’s cognitive computing system that defeated Ken Jennings and Brad Rutter in a game show called Jeopardy! It was the first widely seen demonstration of cognitive computing, and Watson’s ability to answer subtle, complex, pun-laden questions made clear that a new era of computing was at hand.
On the back of that very public success, in 2011, IBM turned Watson toward one of the most lucrative but untapped industries for AI: healthcare. Over the next decade, what followed was a series of ups and downs – but primarily downs – that exemplified the promise and the numerous shortcomings of applying AI to healthcare. The Watson health odyssey finally ended in 2022 when it was sold off “for parts.”
In retrospect, IBM described Watson as a learning journey for the company. “There have been wrong turns and setbacks,” IBM says, “but that comes with trying to commercialize pioneering technology.”
Fast forward to today, and IBM is hoping to take advantage of the boom in generative AI technologies that can write human-like text more than a decade after Watson failed to gain market traction. Mukesh Khare, general manager of IBM Semiconductors, told Reuters recently that one of the old Watson system’s barriers was high costs, which IBM hopes to address now.
YOU MIGHT LIKE
New AI Chips Rule Further Sours US Tech Sales
Khare said using its own chips could lower cloud service costs because they are power efficient.
On the AI chip
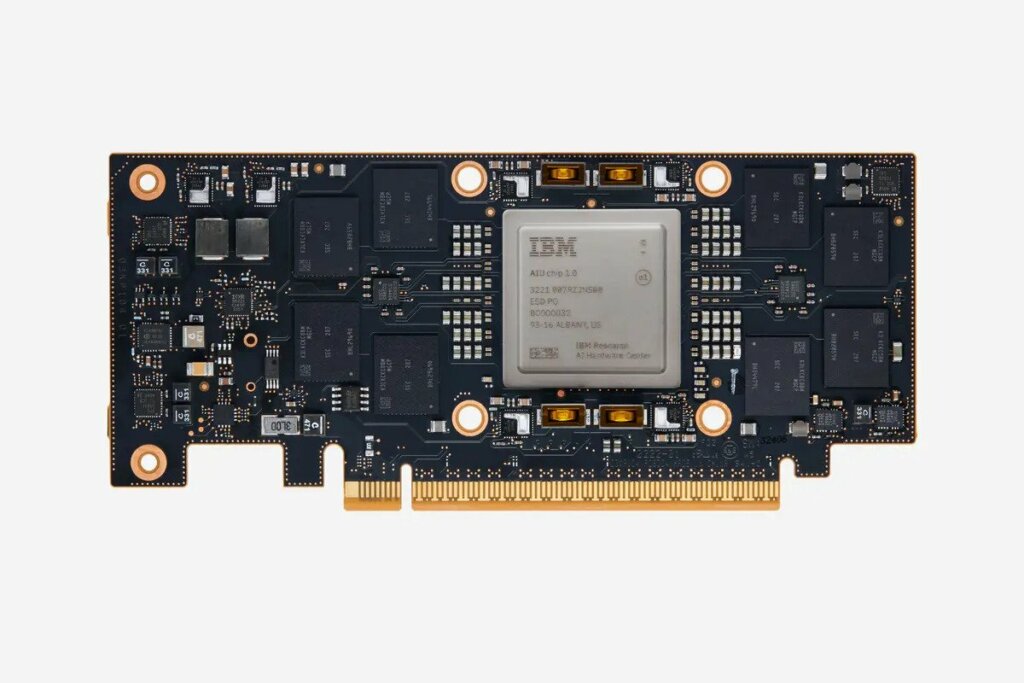
IBM Research AI Hardware Center created a specialized computer chip for AI – calling it an Artificial Intelligence Unit, or AIU. Source: IBM
IBM announced the chip’s existence in October 2022, but did not disclose the manufacturer or how it would be used. “It’s our first complete system-on-chip designed to run and train deep learning models faster and more efficiently than a general-purpose CPU,” IBM said in a release dated October 13, 2022.
IBM also shared that, for the last decade, it had run deep learning models on CPUs and GPUs — graphics processors designed to render images for video games — when it needed an all-purpose chip optimized for the types of matrix and vector multiplication operations used for deep learning. “At IBM, we’ve spent the last five years figuring out how to design a chip customized for the statistics of modern AI,” it said.
IBM was trying to say that AI models are growing exponentially, but the hardware to train these behemoths and run them on servers in the cloud or on edge devices like smartphones and sensors hasn’t advanced as quickly. “That’s why the IBM Research AI Hardware Center created a specialized computer chip for AI. We’re calling it an Artificial Intelligence Unit, or AIU,” the tech giant iterated.
The workhorses of traditional computing — standard chips known as CPUs — were designed before the revolution in deep learning, a form of machine learning that makes predictions based on statistical patterns in big data sets. “CPUs’ flexibility and high precision suit general-purpose software applications. But those winning qualities put them at a disadvantage when training and running deep learning models, which require massively parallel AI operations,” IBM added.
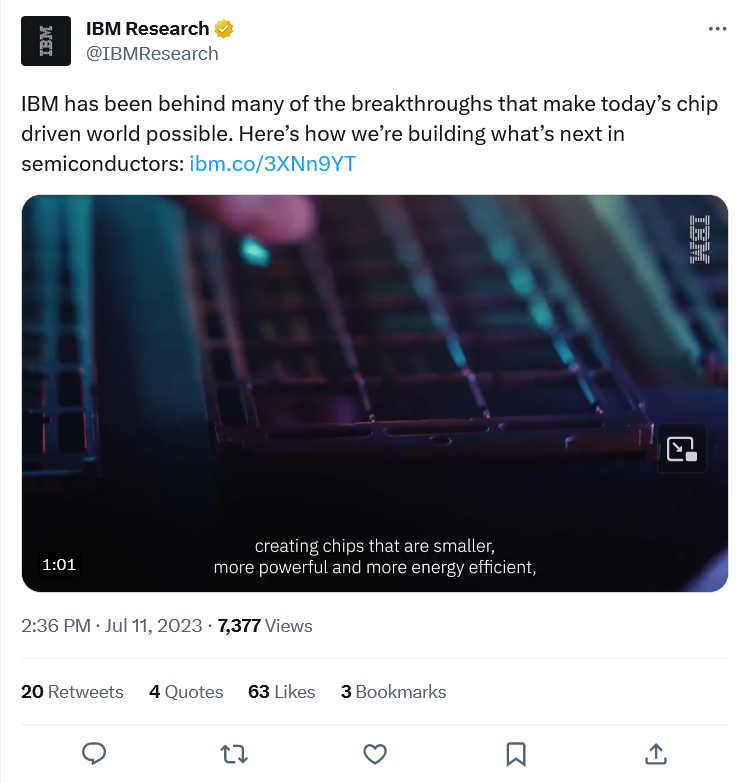
Can an in-house AI chip gamble pay dividends for IBM?
The AI chip by the tech giant uses a range of smaller bit formats, including both floating point and integer representations, to make running an AI model far less memory intensive. “We leverage key IBM breakthroughs from the last five years to find the best trade-off between speed and accuracy,” the company said.
So by leveraging its own chips, IBM aims to improve cost efficiency, which could make their cloud service more competitive in the market. Khare also told Reuters that IBM has collaborated with Samsung Electronics for semiconductor research and has selected it to manufacture those AI chips.
The approach is similar to those adopted by other tech giants like Google and Amazon. IBM, too, by developing proprietary chips, can differentiate its cloud computing service in the market. But Khare reassured the market that IBM was not trying to design a direct replacement for semiconductors from Nvidia, whose chips lead the market in training AI systems with vast amounts of data.